9 1 Setting Up a Factorial Experiment Research Methods in Psychology
Table Of Content
- 2. Multiple Independent Variables¶
- The Past, Present and Future of Factorial Survey Experiments: A Review for the Social Sciences
- Nonmanipulated Independent Variables
- 3.7. Interactions¶
- 1.2. Measures of Different Constructs¶
- Mixed Factorial Designs: Analysis and Interpretation
- Factorial Survey Experiments
- Setting Up a Factorial Experiment
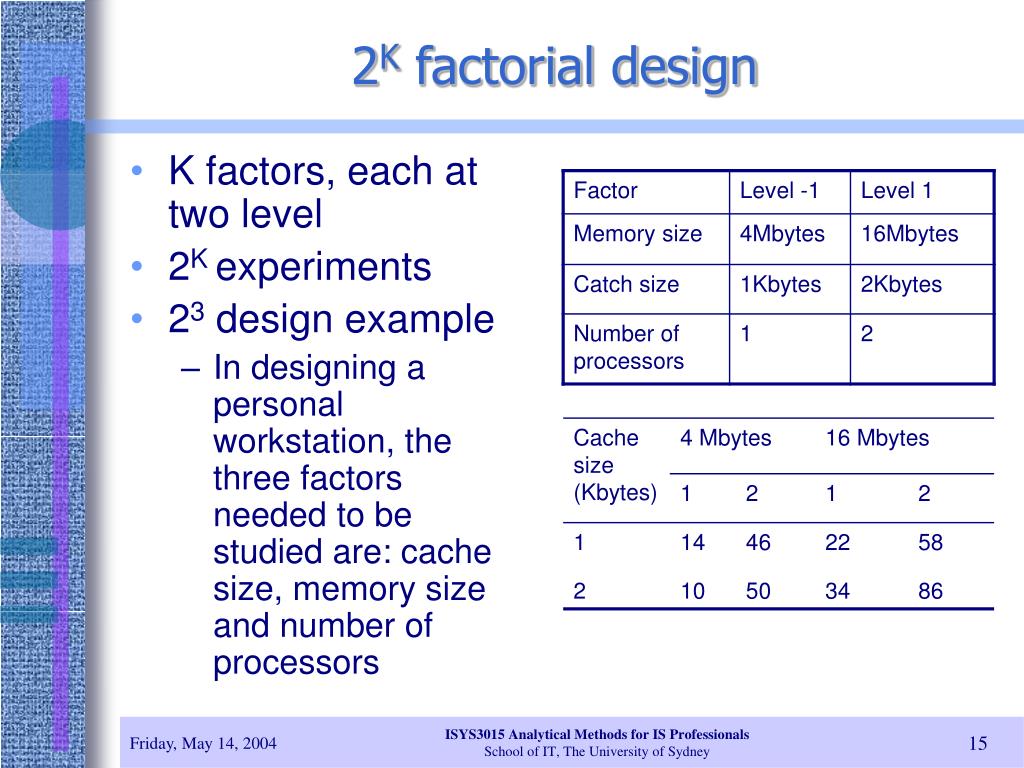
For example, it is possible to use qualitative data to illustrate a quantitative effect, or to determine whether the qualitative and the quantitative component yield convergent results (triangulation). An integrated result could also consist of a combination of a quantitatively established effect and a qualitative description of the underlying process. In the case of development, integration consists of an adjustment of an, often quantitative, for example, instrument or model or interpretation, based on qualitative assessments by members of the target group.
Phosphorus removal and recovery from wastewater via hybrid ion exchange nanotechnology: a study on sustainable ... - Nature.com
Phosphorus removal and recovery from wastewater via hybrid ion exchange nanotechnology: a study on sustainable ....
Posted: Thu, 21 Jan 2021 08:00:00 GMT [source]
2. Multiple Independent Variables¶
This can be conceptualized as a 2 × 2 factorial design with mood (positive vs. negative) and self-esteem (high vs. low) as non-manipulated between-subjects factors. But factorial designs can also include only non-manipulated independent variables, in which case they are no longer experiments but are instead non-experimental (cross-sectional) in nature. But factorial designs can also include only non-manipulated independent variables, in which case they are no longer experiment designs, but are instead non-experimental in nature. In a between-subjects factorial design, all of the independent variables are manipulated between subjects.
The Past, Present and Future of Factorial Survey Experiments: A Review for the Social Sciences
Main effects occur when the levels of an independent variable cause change in the measurement or dependent variable. There is one possible main effect for each independent variable in the design. When we find that independent variable did influence the dependent variable, then we say there was a main effect. When we find that the independent variable did not influence the dependent variable, then we say there was no main effect. When researchers combine dependent variables in this way, they are treating them collectively as a multiple-response measure of a single construct. The advantage of this is that multiple-response measures are generally more reliable than single-response measures.
Nonmanipulated Independent Variables
As we have already seen, researchers conduct correlational studies rather than experiments when they are interested in noncausal relationships or when they are interested variables that cannot be manipulated for practical or ethical reasons. In this section, we look at some approaches to complex correlational research that involve measuring several variables and assessing the relationships among them. The second way of looking at the interaction is to start by looking at the other variable.
3.7. Interactions¶
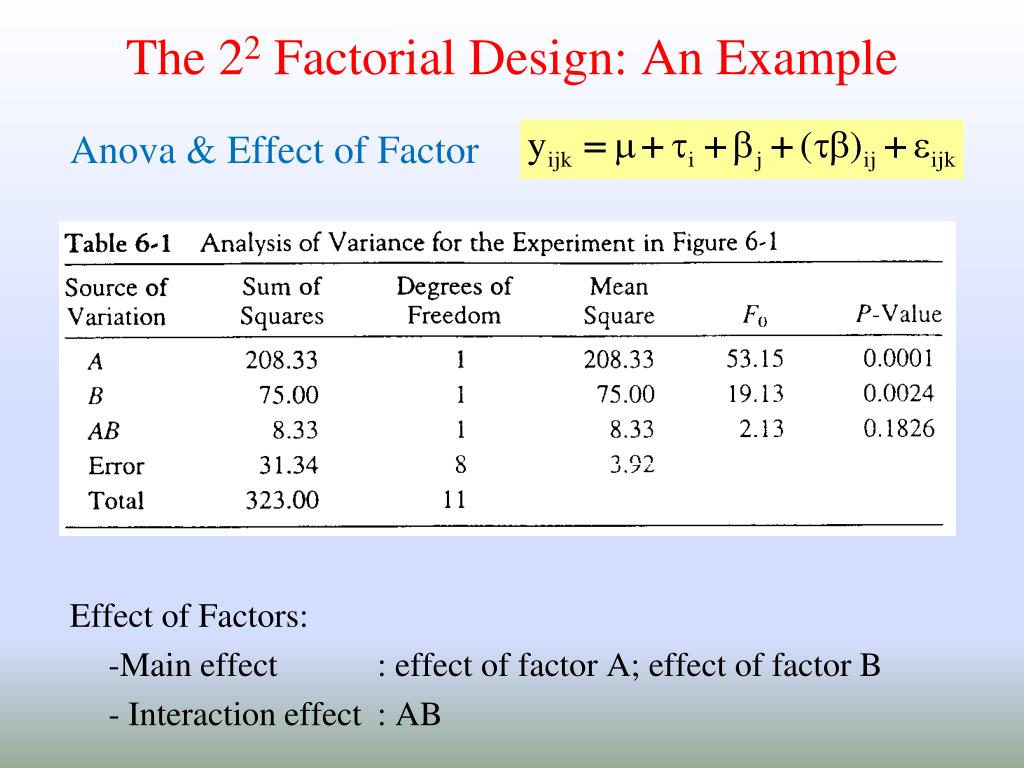
In many factorial designs, one of the independent variables is a non-manipulated independent variable. The other was private body consciousness, a variable which the researchers simply measured. Another example is a study by Halle Brown and colleagues in which participants were exposed to several words that they were later asked to recall [BKD+99].
1.2. Measures of Different Constructs¶
The interaction suggests that something special happens when people are tired and haven’t eaten in 5 hours. Whereas, in the other conditions, there are only small increases in being hangry. An advantage of these graphs is that they display means in all four conditions of the design. Someone looking at this graph alone would have to guesstimate the main effects. If we made a separate graph for the main effect of shoes we should see a difference of 1 inch between conditions. Similarly, if we made a separate graph for the main effect of hats then we should see a difference of 6 between conditions.
The red bars show the conditions where people wear hats, and the green bars show the conditions where people do not wear hats. For both levels of the wearing shoes variable, the red bars are higher than the green bars. More specifically, in both cases, wearing a hat adds exactly 6 inches to the height, no more no less. The primary design dimensions explained above have been the focus of this article. There are a number of secondary considerations for researchers to also think about when they design their studies (Johnson and Christensen 2017). Now we list some secondary design issues and questions that should be thoughtfully considered during the construction of a strong mixed methods research design.
When designing a mixed methods study, it is sometimes helpful to list the purpose in the title of the study design. For this example, we will now consider both the between-subjectfactor of age, as well as the within-subject factor of condition. Do tothis, we will average over the different trials (1, 2, 3, and 4) to getone observation in each condition. This design is sometimes referred asa “mixed-factorial” design, because we have a mix of between-subjectsand within-subject factors. Depending on your background, you might bemore familiar with this as a “split plot” design.
Setting Up a Factorial Experiment
We can construct another design using this component as our generator to confound with blocks. Let's now look at the one replicate where we will confound the levels of the AB component with our blocks. We will label these 0, 1, and 2 and we will put our treatment pairs in blocks from the following table. We will take one replicate of this design and partition it into 3 blocks.
Before we do, let’s consider the analysis of variance table for this single replicate of the design. Next, we will consider the more complicated example of our fullyfactorial design with a nested factor of Age-Group, and crossed factorsof Condition and Time. For this mixed factorial ANOVA, we have one factor of Condition thatvaries within-subjects, but we also have a factor of Age Group thatvaries between subjects.
For more information on how to articulate design complexity based on multiple purposes of mixing, see Schoonenboom et al. (2017). Ultimately, mixed methods research is about heightened knowledge and validity. In a commonly used mixed methods notation system (Morse 1991), the components are indicated as qual and quan (or QUAL and QUAN to emphasize primacy), respectively, for qualitative and quantitative research. Note that each research tradition receives an equal number of letters (four) in its abbreviation for equity. However, we can also perform a two-way ANOVA to formally test whether or not the independent variables have a statistically significant relationship with the dependent variable. When one independent variable is manipulated between subjects and another is manipulated within subjects.
Comments
Post a Comment